Discover our blog
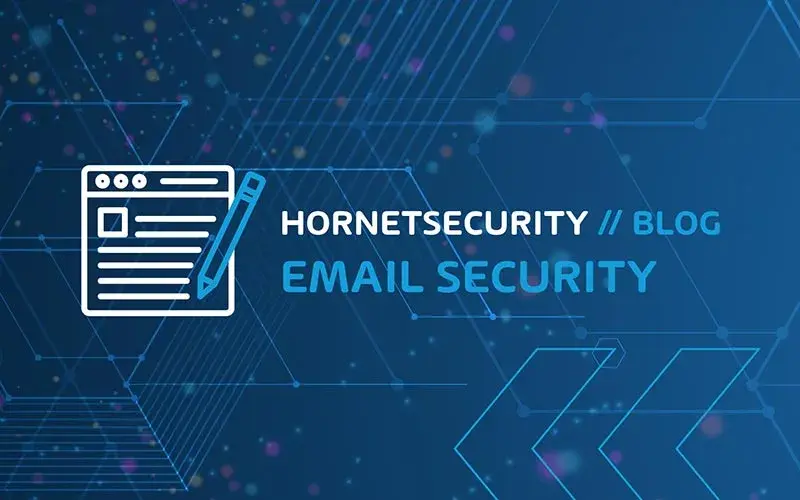
Email Security
Hornetsecurity and Vade Email Filtering – Stronger Together
By Paul Schnackenburg, on September 26, 2024
Vade joining the Hornetsecurity group has brought big benefits to customers from both sides, with the combination of...